Table of Contents
- Potential Security Risks of AI, ML, and IoT in Enterprise Networks
- How Edge Computing and AI Impact Security and Reliability
- Best Practices for Securing IoT, Edge, and AI-Enabled Devices
- Protecting the Future of AI and IoT in Enterprise Networks
Internet of Things (IoT) devices have transformed enterprise networks, making operations more efficient and data-driven. However, as businesses rely more on connected devices, they face growing security and reliability risks. Each sensor, actuator, camera, and machine learning-enabled system represents a potential entry point for attackers. AI-enabled devices process vast amounts of sensitive data, make autonomous decisions, and interact with cloud and edge environments. If left unprotected, they introduce risks that go beyond traditional cybersecurity threats. As AI and edge computing become central to enterprise infrastructure, these risks only increase, posing new enterprise security challenges.
Traditional models for enterprise security were not designed for networks of thousands of constantly communicating endpoints. To protect their networks, enterprises need to rethink security strategies. Businesses must secure not only the devices themselves but also the models, data pipelines, and decision-making processes that drive them.
Potential Security Risks of AI, ML, and IoT in Enterprise Networks
Many IoT devices prioritize functionality over security, often shipping with default credentials, unencrypted data transmission, and limited firmware updates. Attackers take advantage of these weaknesses to gain unauthorized access, move laterally through networks, and extract valuable data. AI-enabled IoT devices add another layer of complexity because they process and act on information in real time. If an attacker manipulates the data an AI model relies on, it can alter decision-making processes in ways that are difficult to detect.
One emerging threat is data poisoning, where attackers feed manipulated data into an AI system to influence its behavior. This can lead to security blind spots, such as an AI-based threat detection system failing to recognize an attack. Another risk is model extraction, where an adversary reverse-engineers an AI model to understand how it makes decisions, allowing them to craft more effective attacks. Because AI models often rely on third-party frameworks, enterprises must also be cautious about vulnerabilities in the software supply chain. If a compromised AI model is deployed in a security-sensitive environment, attackers could introduce backdoors without detection.
Model drift presents a more subtle but equally significant challenge. Over time, an AI model’s accuracy can degrade as real-world conditions change. A security system trained on past threats may not recognize new attack patterns, leaving networks exposed. Without ongoing monitoring and retraining, businesses may not realize their AI defenses have become ineffective.
Machine learning (ML) models introduce another risk: adversarial attacks. These attacks manipulate input data in ways that fool ML algorithms into making incorrect classifications. For example, an attacker could alter sensor data in a way that causes an AI security system to ignore a real threat. Unlike traditional cyber threats, adversarial attacks are designed to exploit the mathematical structure of ML models, making them harder to detect.
AI-driven enterprise security solutions provide valuable automation and threat detection capabilities, but they are not foolproof. If an AI system misclassifies normal network activity as a threat, it could disrupt legitimate operations. Conversely, if attackers exploit weaknesses in AI-based detection, they may be able to bypass security measures altogether. To mitigate these risks, enterprises should combine AI-powered security with traditional rule-based approaches and maintain human oversight where possible.
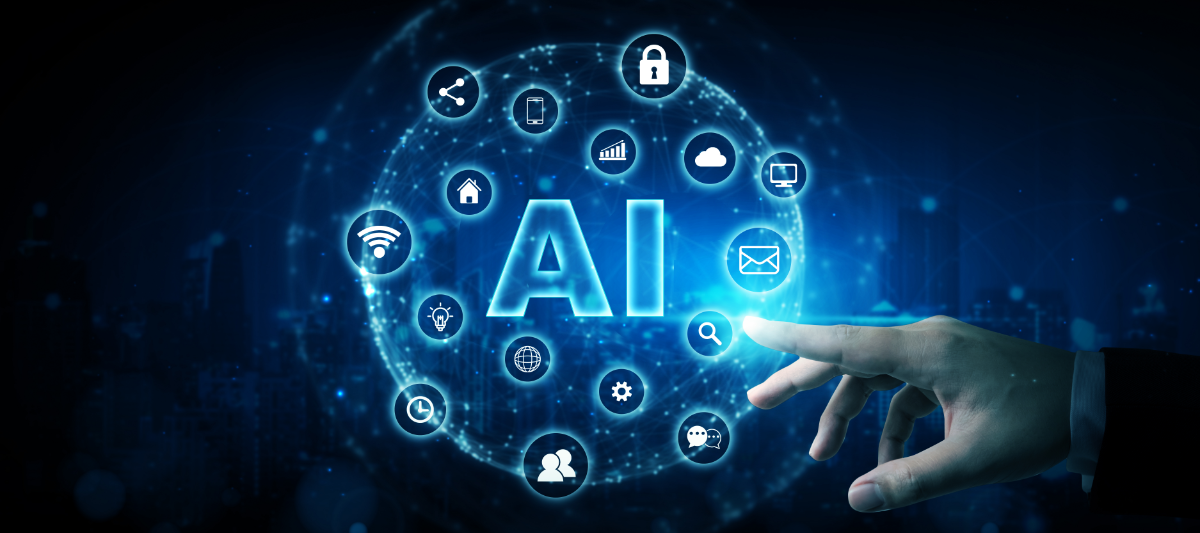
How Edge Computing and AI Impact Security and Reliability
Edge computing reduces the need to send all IoT data to the cloud, improving both speed and security. By processing information closer to the source, edge devices reduce latency and minimize exposure to cyber threats during data transmission. But securing edge deployments comes with challenges. Unlike traditional data centers, edge computing often distributes nodes across multiple locations, making centralized security controls less effective. Teams must secure, monitor, and update each edge device to prevent vulnerabilities.
ML-enabled edge devices bring additional risks. These devices make real-time decisions without human oversight, which is useful for applications like automated threat detection and response. However, if an AI model misclassifies a threat, it could block legitimate traffic or fail to stop an actual attack. To prevent this, enterprises should implement human-in-the-loop (HITL) validation, ensuring that AI-driven security decisions undergo periodic review.
AI-based self-healing networks offer another advantage. These systems use ML models to predict network failures and automatically reroute traffic to maintain reliability. However, attackers who manipulate ML models could disrupt this process, causing unnecessary reconfigurations or network congestion. Businesses can improve reliability by segmenting AI-powered devices from critical infrastructure, using federated learning to retrain models locally, and applying explainable AI (XAI) techniques to understand AI-driven decisions better.
Best Practices for Securing IoT, Edge, and AI-Enabled Devices
A Zero Trust approach is essential for securing IoT and AI devices. Systems must authenticate every device, application, and user before allowing access to enterprise resources. Strong encryption and secure authentication protocols, such as public key infrastructure (PKI) and multifactor authentication (MFA), help prevent unauthorized access.
Businesses should also establish network segmentation to prevent lateral movement in the event of a breach. Keeping IoT and AI-powered devices in separate network zones limits the potential impact of an attack. Microsegmentation goes even further by restricting device-to-device communication based on predefined policies.
Regular updates and patch management are critical. Many cyberattacks exploit outdated software or unpatched vulnerabilities. Where possible, enterprises should deploy automated update systems to ensure IoT and AI devices receive security patches without manual intervention.
AI governance plays an increasingly vital role in security strategy. Enterprises should audit AI models for bias, accuracy, and adversarial vulnerabilities. XAI techniques help security teams understand why an AI system flagged a particular activity as suspicious, reducing false positives and improving response times.
To complement AI-driven threat detection, IT staff should ensure human oversight. Security teams should continuously monitor AI-based alerts, validating their accuracy and adjusting detection models when needed. Combining AI and human expertise provides a more resilient security framework.
Protecting the Future of AI and IoT in Enterprise Networks
AI and edge computing have the potential to make enterprise networks smarter, more efficient, and more secure. However, without the right protections, they also introduce new attack surfaces and operational risks. Enterprises must move beyond traditional security models and adopt proactive, AI-aware cybersecurity strategies.
At Sparro, we help enterprises deploy secure, high-performance networks that support IoT, edge computing, and AI-driven applications. Whether you need advanced threat detection, network segmentation, or AI-powered security solutions, our technology ensures your infrastructure remains both protected and reliable.
Want to learn more about protecting your IoT- and AI-enabled network? Contact us today.